New evidence that quantum machine learning outperforms classical computing
April 5, 2023
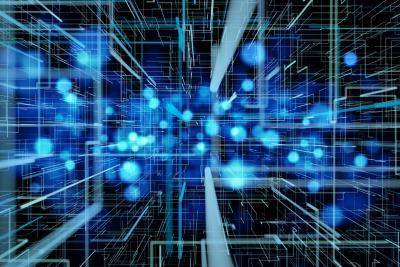
April 5, 2023
Quantum machine learning models can achieve ‘quantum advantage’ by solving a complex class of mathematical problems impossible to crack with a classical computer, according to new research by UBC material scientists.
UBC Blusson Quantum Mater Institute (Blusson QMI) investigator Professor Roman Krems said the results rigorously prove that quantum machine learning does indeed offer the quantum advantage.
“The key goal now is to find a real-world machine learning application that would benefit from this quantum advantage in practice,” said Professor Krems, senior author on the Nature Communications study.
Quantum advantage refers to the instances where quantum computers outperform their classical counterparts when scaling to enormous datasets containing countless variables.
Blusson QMI PhD student and first author of the paper Jonas Jäger said the models have universal expressiveness in that they solve not just one problem, but capture the complexity of an entire class of problems that are too complicated to solve with classical machine learning.
“While quantum machine learning is often considered to be one of the most promising use cases of quantum computing, there are only a few rigorous results about its real computational advantages,” Jäger said. “Our results offer theoretical guarantees that such advantages indeed exist.”
The study proves a quantum advantage exists for two of the most popular quantum machine learning classification models: Variational Quantum Classifiers (also known as quantum neural networks) and Quantum Kernel Support Vector Machines.
“We can now confidently explore important real-world applications and develop effective approaches for building informative data encoding quantum circuits that could unlock the full potential of quantum machine learning,” said Jäger.
The advantages reported in the study are somewhat subject to the quality of the datasets presented to the system. As quantum computing is still in the experimental stage, a challenge faced by researchers is encoding the classical data for processing by a quantum device.
“The mathematical problem that we’ve solved using these models is quite abstract and doesn’t have many practical applications. But, because it presents such special properties under the complexity theory, it can be used by others as a benchmark to test how different quantum machine learning models perform,” Jäger said.
Jäger joined UBC in Sept 2022 to commence his PhD studies under the supervision of Professor Roman Krems from UBC’s Department of Chemistry and Professor Michael Friedlander from UBC’s Computer Science Department.
Professor Krems and his team work at the intersection of quantum physics, machine learning and chemistry on problems of relevance to quantum materials and quantum technologies, including quantum computing, quantum sensing and quantum algorithms. Meanwhile, Professor Friedlander and his research group develop theories and algorithms for mathematical optimization and its applications in machine learning, signal processing and operations research.
Jäger hopes to take advantage of their combined expertise to push the limits of quantum computing and develop algorithms that can harness its power for practical applications.
We honour xwməθkwəy̓ əm (Musqueam) on whose ancestral, unceded territory UBC Vancouver is situated. UBC Science is committed to building meaningful relationships with Indigenous peoples so we can advance Reconciliation and ensure traditional ways of knowing enrich our teaching and research.
Learn more: Musqueam First Nation